Meta has been training on its new Llama 3 405B model on a cluster of 16,384 x NVIDIA H100 80GB AI GPUs. Half of the issues during its 54-day training run were caused by the onboard HBM3 memory.
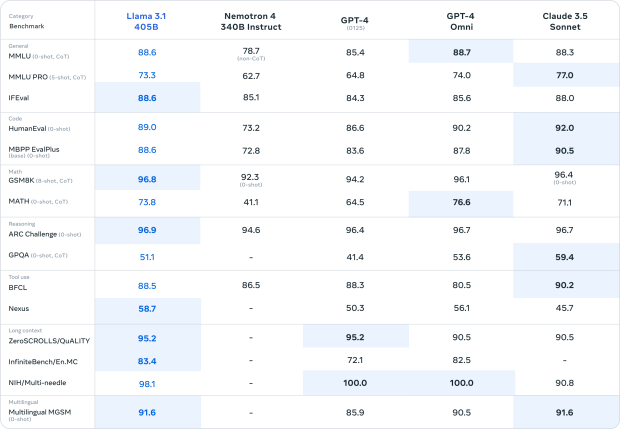
Meta released a new study detailing its Llama 3 405B model training, which took 54 days with the 16,384 NVIDIA H100 AI GPU cluster. During that time, 419 unexpected component failures occurred, with an average of one failure every 3 hours. In half of those failures, GPUs or their onboard HBM3 memory were the blame.
In a system with truck loads of components like CPUs, motherboards, RAM, SSDs, GPUs, power systems, cooling systems, a supercomputer is exotic and ultimately powerful, but it's completely normal for issues to happen every few hours. But, it's how developers work on those issues and get the system to remain operational no matter what local breakdowns are happening.
For a gigantic cluster of 16,384 AI GPUs you're bound to run into issues, but if a single GPU goes down then that can disrupt the entire AI training job and when they're running for 54 days, the notion that you'd have to start again would make for some sleepiness nights. With all of those AI GPUs running in a cluster, the Llama 3 team maintained over a 90% effective training time.
- Read more: Meta releases 'world's largest' AI model trained on $400 million worth of GPUs
- Read more: Meta has two new AI data centers equipped with over 24,000 NVIDIA H100 GPUs
- Read more: Meta's long-term vision for AGI: 600,000 x NVIDIA H100-equivalent AI GPUs for future of AI
In the 54-day pre-training snapshot, the Meta team noted 466 job interruptions, with 47 planned and 419 unexpected. Planned interruptions were due to automated maintenance, with unexpected issues related to hardware problems. GPU issues accounted for 58.7% of expected interruptions, with just 3 issues requiring significant manual intervention, while the rest were automatically managed.
Out of the 419 unexpected problems, 148 (30.1%) of those were from various GPU failures (including NVLink issues), with 72 (17.2%) being caused by HBM3 memory failures. NVIDIA's current-gen H100 AI GPUs consume around 700W of power, and under considerable thermal stress, which explains some of these issues.
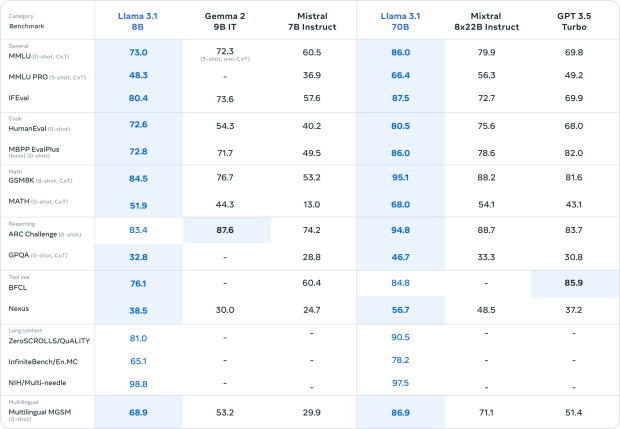
In order to boost efficiency, Meta's team reduced job startup and checkpointing times and created their own proprietary diagnostic tools. PyTorch's NCCL flight recorder was used extensively to quickly find and work through hands and performance problems, especially when it came to NCCLX, which is a tool that captures metadata and stack traces, helping in quick problem resolution.
Straggling GPUs can cause slowdowns to thousands of other GPUs, with the Meta team using its in-house tools to identify specific GPUs with issues. The tools prioritized problematic communications, which means effective detection and the timely resolution of stragglers, making sure that slowdowns are kept to a minimum and maintaining overall training efficiency.
Meta's team noted that mid-day temperature fluctuations, impacted training performance by 1-2% variation throughout testing, with dynamic voltage and frequency scaling of the AI GPUs were affected through these very slight temperature changes, but it wasn't a big problem.
The Llama 3 405B LLM training team expected another issue with simultaneous power consumption changes of tens of thousands of AI GPUs, stressing the power grid inside of the data center. These fluctuations, which can be as high as tens of megawatts, pushed the grid to its limit, making Meta ensure its data centers had enough power.
- Read more: Elon Musk's new Memphis Supercluster uses gigantic portable power generators, grid isn't enough
Meta has a cluster of just 16,384 H100 AI GPUs while Elon Musk with his xAI supercomputer featuring 100,000 H100 AI GPUs, which explains why he's got some seriously powerful portable power generators powering his AI cluster.